
The problem seems trivial, but involves several computer engineering challenges and then the ultimate wall that protein design campaigns usually hit: synthesizing the predicted proteins experimentally and verifying that they truly fold as expected, and even better if they perform the expected function. This group, also developer of protein modeling programs such as RoseTTAFold (less known than AlphaFold but apparently almost as accurate) quickly saw how the new machine learning technologies aimed at predicting protein structures could be reversed to predict which sequences would fold as desired. Without any doubt, the leader group in the domain is the Baker lab at the University of Washington in Seattle, which is actually running a whole Institute for Protein Design. So far, while subproblems such as stabilizing existing proteins are increasingly addressed through machine learning, the problem of creating a whole new protein sequence from scratch has been treated mainly through physics-based methods. This problem is in general coined protein design it has several goal-specific sub-problems of which creating a whole protein from scratch is the hardest. It is very often interesting to tackle the opposite problem: given a function that should be achieved by a given 3D structure (or given any other trait that one wants to optimize, such as stability), what protein sequence do we need (or what mutations on a starting sequence)? (For the biologists: I’m leaving aside the whole other universe of intrinsically disordered proteins.)

Protein structure and protein designĪs I have covered in previous articles on AlphaFold and protein modeling (see an index of them here), protein sequences dictate how a protein will acquire a 3D structure (the fold) which in turn dictates what functions it can exert, as well as its stability, solubility, etc. And eventually this tool, called ProteinMPNN, came out, with which scientists can now design proteins that fold (and hence work) as they need.ĬolabFold and even web app versions of ProteinMPNN are already online for everybody to use. This by itself didn’t turn out to work quite well, but it inspired further strategies for machine learning-based protein design.
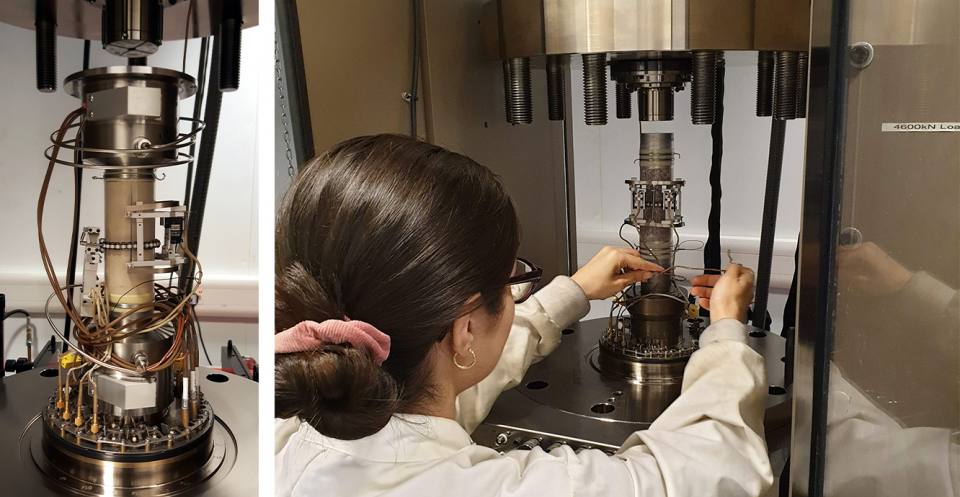
Reverse an AlphaFold-like neural network to feed it 3D structures and obtain from it protein sequences that fold accordingly. But honestly I didn’t expect it to happen so quickly:

This was going to happen, and I expected the Baker lab to be the first group to report it. And you can use it to design your own proteins too, right online.
#CORE DATA LAB METCHODS SOFTWARE#
This new software from the Baker laboratory designs proteins that actually work in the wet lab.
